Introduction:
In recent years, the rapid advancement of artificial intelligence (AI) technologies has revolutionized many industries, including healthcare, finance, education, and retail. AI’s ability to process vast amounts of data and make decisions based on patterns has enabled businesses to offer more personalized services and streamline operations. However, with the widespread use of AI, one critical concern has emerged: how can we ensure that data privacy is safeguarded in an increasingly data-driven world?
Data privacy has become a paramount issue as individuals’ personal information is continuously being collected, analyzed, and stored by AI systems. This information can include anything from basic personal details to sensitive data, such as health records or financial transactions. With AI systems handling and processing large volumes of personal data, it is essential to understand the potential risks and the ways in which data privacy can be effectively protected.
This article will explore the challenges AI poses to data privacy, the regulations and technologies available to safeguard privacy, and the steps that can be taken to ensure data protection in the age of AI.
1. The Rising Importance of Data Privacy in the Age of AI
As AI technology becomes more sophisticated, it has increasingly been able to analyze and predict individual behavior with remarkable accuracy. AI’s reliance on massive datasets, including personal and behavioral data, means that data privacy concerns are becoming more prominent. The combination of AI and big data creates the potential for a breach of privacy, making it crucial to examine the extent to which personal data is being collected, how it is used, and who has access to it.
1.1. AI’s Role in Data Collection and Analysis
AI systems thrive on data, and many of the most successful AI applications, such as personalized recommendations, voice assistants, and predictive algorithms, are built on a foundation of personal data. AI models are trained using datasets that often include sensitive information, such as health status, location, purchase history, and social interactions. The more granular the data, the more accurate and effective the AI system becomes.
However, this raises concerns about the breadth of data being collected and the potential misuse of that data. For instance, AI-powered surveillance systems could monitor individuals’ activities on a vast scale, including their online behaviors, movements, and even personal interactions, leading to significant privacy risks.
1.2. Privacy Violations and Data Exploitation
With the increasing capabilities of AI to analyze and predict behaviors, there is a growing risk of privacy violations. AI systems could inadvertently expose private information, use it in ways that the individual did not consent to, or allow third parties to access sensitive data without proper safeguards. Furthermore, companies may exploit users’ data for commercial gain without adequate transparency or accountability.
These privacy risks highlight the urgent need for stringent data privacy protections to ensure that personal data remains secure and is used responsibly.
2. Challenges to Data Privacy Posed by AI
The integration of AI into everyday life presents several challenges to data privacy, particularly due to the nature of AI systems and how they function. Some of these challenges include:
2.1. Data Minimization and Informed Consent
One fundamental principle of data privacy is that organizations should only collect the minimum amount of personal data necessary for a given purpose (data minimization). However, AI systems often require extensive datasets to function effectively, sometimes collecting far more data than is necessary. This can lead to over-collection of personal information, making it difficult for individuals to maintain control over their data.
Furthermore, ensuring that individuals provide informed consent for the use of their data is another critical challenge. As AI systems become more complex, it becomes increasingly difficult for users to fully understand how their data is being used and what potential risks they are exposing themselves to. AI technologies often operate as “black boxes,” meaning that even those who create and deploy AI models may not fully understand how the algorithms process data, raising concerns about transparency and accountability.
2.2. Bias and Discrimination
AI systems rely on data to make predictions and decisions, and if that data contains biases, these biases can be reinforced and even amplified by the AI. For example, facial recognition systems may have higher error rates for people of certain ethnicities, leading to discrimination and potential violations of privacy.
In addition, AI systems trained on biased or incomplete datasets can lead to unfair outcomes, such as denying individuals access to critical services or opportunities based on skewed data. Ensuring that AI systems are designed to mitigate bias is essential for protecting privacy and ensuring that personal data is used fairly and equitably.
2.3. Lack of Accountability
As AI technologies evolve and are integrated into various industries, the question of accountability becomes more pressing. If an AI system causes a privacy breach or makes an erroneous decision based on personal data, it is often unclear who is responsible. Is the responsibility placed on the developer of the AI, the organization using the AI, or the AI system itself? Lack of clear accountability can make it difficult to resolve privacy issues and hold parties responsible for violations.
Furthermore, the difficulty in tracking the decisions made by AI systems—especially those based on machine learning and deep learning—compounds the challenge of ensuring transparency in how personal data is being processed.
3. Regulatory Measures to Protect Data Privacy
As AI continues to play a central role in data-driven applications, governments and regulatory bodies are increasingly introducing laws and regulations to ensure data privacy and security. Some of the most prominent regulations include:
3.1. General Data Protection Regulation (GDPR)
The European Union’s General Data Protection Regulation (GDPR) is one of the most comprehensive data privacy regulations introduced to date. GDPR sets strict guidelines on how organizations must collect, store, and process personal data, including provisions for the right to access, rectify, and erase personal data. Importantly, GDPR also emphasizes the principle of “data protection by design,” which requires organizations to integrate privacy measures into the design of their systems, including AI models.
The GDPR’s impact on AI development has been profound, as it compels companies to be more transparent about how AI systems use personal data. This regulation also gives individuals more control over their data, making it easier for them to opt out of AI-driven services that they feel are intrusive or unnecessary.
3.2. California Consumer Privacy Act (CCPA)
In the United States, the California Consumer Privacy Act (CCPA) represents a significant step toward protecting data privacy in the age of AI. The CCPA grants California residents various rights, including the right to know what personal data is being collected, the right to request that data be deleted, and the right to opt-out of the sale of their data. It also provides provisions for individuals to access and delete data stored by companies, enhancing consumer control over how their information is used by AI-powered systems.
Although the CCPA applies only to California residents, it has set a precedent for similar legislation in other states and may influence future federal data privacy laws in the U.S.
3.3. Artificial Intelligence Ethics Guidelines
In addition to data protection laws, many organizations and governments are developing AI ethics guidelines to address privacy and accountability issues specific to AI technologies. For example, the OECD (Organisation for Economic Co-operation and Development) has established AI principles that promote transparency, accountability, and fairness in AI systems. These guidelines emphasize the importance of privacy protection and advocate for the use of AI in a way that respects human rights and individual freedoms.
As AI technologies continue to evolve, regulatory bodies will likely continue to refine and expand upon these ethical guidelines to address emerging privacy concerns.
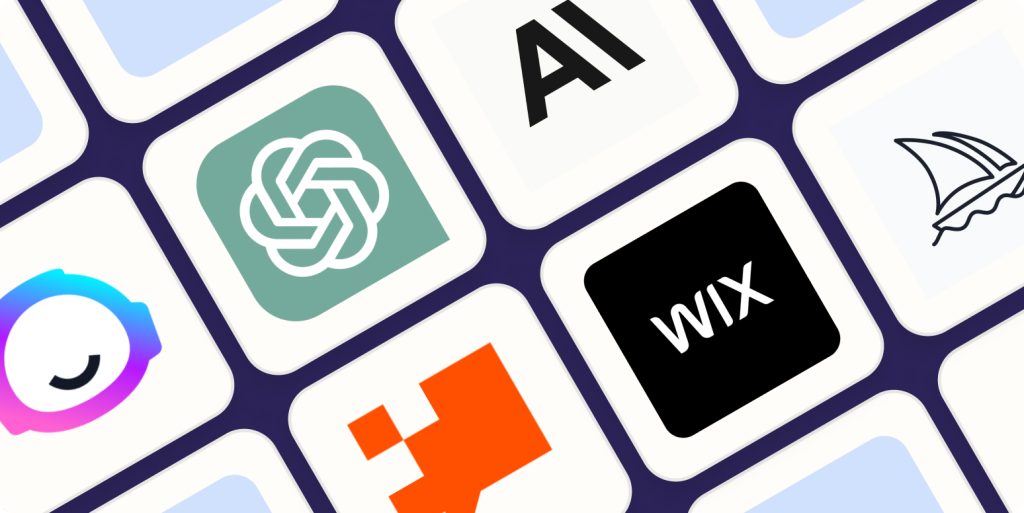
4. Technological Solutions for Safeguarding Privacy in AI
In addition to regulatory measures, various technological solutions are being developed to safeguard data privacy in AI applications. These solutions aim to protect personal data while still allowing AI systems to function effectively.
4.1. Differential Privacy
Differential privacy is a privacy-enhancing technique that ensures the privacy of individual data points within a dataset. By adding controlled noise to data, differential privacy allows organizations to extract valuable insights from datasets while minimizing the risk of exposing individual information. This approach is increasingly being used in AI systems to enable data analysis without compromising privacy.
For example, Apple and Google have implemented differential privacy techniques in their AI systems to analyze user data for improving services without revealing personal information. By embedding differential privacy into AI tools, organizations can better safeguard the privacy of individuals while still benefiting from AI-driven insights.
4.2. Federated Learning
Federated learning is a machine learning approach that allows AI models to be trained across decentralized devices (such as smartphones or computers) without the need to send personal data to a central server. This technique enables AI systems to learn from local data stored on individual devices, which significantly reduces the risks associated with data transfer and central storage.
By keeping sensitive data on users’ devices and only sharing aggregated learning models, federated learning helps protect privacy while still enabling the development of robust AI models. This approach is being adopted in various industries, such as healthcare and finance, where privacy is a top priority.
4.3. Homomorphic Encryption
Homomorphic encryption is an advanced encryption technique that allows computations to be performed on encrypted data without decrypting it. This means that AI systems can process and analyze encrypted data without exposing it, making it highly effective for protecting privacy.
Homomorphic encryption can be used to protect personal data in AI applications, especially in sensitive areas like healthcare or finance, where data privacy is paramount. By enabling AI to operate on encrypted data, this technology allows for secure data processing while ensuring that sensitive information remains confidential.
5. Best Practices for Ensuring Data Privacy in AI
To effectively safeguard data privacy in AI, both organizations and individuals must adopt best practices that prioritize privacy protection. These practices include:
5.1. Implementing Privacy by Design
Organizations should embed privacy considerations into the design and development of AI systems from the outset. This approach, known as “privacy by design,” ensures that privacy protections are built into every stage of the AI system’s lifecycle, from data collection to processing and storage.
5.2. Transparency and Consent
AI systems should be transparent about how data is collected and used. Organizations must inform users about the types of data being collected, the purpose of the data collection, and how their data will be used. Obtaining explicit consent from individuals before collecting or processing their data is essential for ensuring privacy and trust.
5.3. Regular Audits and Monitoring
To ensure compliance with privacy laws and regulations, organizations should conduct regular audits of their AI systems to assess how data is being used and whether privacy risks are being mitigated. Continuous monitoring and updating of AI systems can help identify potential privacy issues before they become serious problems.
Conclusion:
As artificial intelligence continues to advance, data privacy will remain one of the most critical issues facing society. AI systems that process vast amounts of personal data can offer significant benefits, but they also pose serious risks to privacy. To safeguard data privacy, it is essential to implement robust regulatory frameworks, adopt privacy-enhancing technologies, and ensure transparency and accountability in AI systems.
By taking proactive steps to protect privacy, both through legal measures and technological innovations, we can harness the power of AI while maintaining the fundamental rights of individuals to control their personal data. In this way, we can create a future where AI and data privacy coexist harmoniously, benefiting society without compromising individual rights.