Introduction
The logistics and transportation industry is facing growing challenges as the demand for faster deliveries, reduced costs, and more efficient operations continues to rise. Traditional methods of managing supply chains, optimizing delivery routes, and keeping track of inventory can no longer meet the demands of modern businesses. In response, Artificial Intelligence (AI) has emerged as a game-changer, offering solutions that significantly enhance operational efficiency.
AI technologies are being applied in various aspects of logistics and transportation, with route planning and inventory management being two key areas that have seen transformative changes. From minimizing delivery times to maximizing the use of warehouse space, AI is streamlining processes, improving decision-making, and reducing operational costs.
This article delves into how AI is optimizing route planning and inventory management within the logistics and transportation industry, providing practical insights and case studies from real-world applications.
Section 1: The Role of AI in Logistics and Transportation
1.1 The Evolution of Logistics and Transportation
Logistics and transportation have always been critical to global commerce. However, with the increasing complexity of supply chains and the rise of e-commerce, the industry is experiencing a shift. Companies need faster delivery, more accurate inventory tracking, and better resource utilization. AI helps tackle these challenges by processing large volumes of data quickly, optimizing decision-making, and automating repetitive tasks.
1.2 AI Technologies Impacting Logistics and Transportation
Several AI technologies are driving innovation in logistics and transportation:
- Machine Learning (ML): Used to analyze data and make predictions based on historical trends, such as predicting traffic patterns or demand surges.
- Computer Vision: Applied in warehousing for identifying and sorting products, as well as in autonomous vehicles for navigation and obstacle avoidance.
- Natural Language Processing (NLP): Helps in automating customer service inquiries and streamlining communication between systems.
- Robotics and Automation: Used for handling goods in warehouses, minimizing human error, and speeding up sorting processes.
Section 2: AI in Route Planning Optimization
2.1 What is Route Planning in Logistics?
Route planning involves determining the most efficient path for transporting goods from one location to another. Traditional route planning relied on predefined maps and manual adjustments, but these methods could not adapt to real-time conditions. AI revolutionizes this by utilizing data from various sources to optimize routes dynamically.
2.2 How AI Optimizes Route Planning
AI leverages vast amounts of real-time data to improve route planning. By integrating traffic conditions, weather reports, historical data, and other factors, AI algorithms can suggest the best possible routes for delivery trucks. This not only reduces travel time but also cuts fuel costs, improves delivery reliability, and enhances customer satisfaction.
Key AI Tools for Route Optimization:
- Real-Time Traffic Analysis: AI can predict traffic patterns based on historical data and real-time inputs like road closures and accidents, helping companies avoid delays.
- Dynamic Routing: AI algorithms continuously update routes based on real-time conditions, ensuring that drivers always follow the quickest path.
- Fuel Efficiency Models: AI models can calculate the most fuel-efficient routes, reducing the environmental impact and lowering transportation costs.
2.3 Case Study: UPS – ORION System
United Parcel Service (UPS) has implemented AI in its operations to optimize its delivery routes through a system called ORION (On-Road Integrated Optimization and Navigation). ORION uses real-time data to analyze traffic conditions, weather patterns, and package locations to determine the most efficient delivery route. By using ORION, UPS has saved millions of dollars annually and reduced its carbon footprint by eliminating unnecessary travel.
Lessons Learned:
- The importance of real-time data in route optimization.
- How AI can reduce fuel consumption and increase overall operational efficiency.
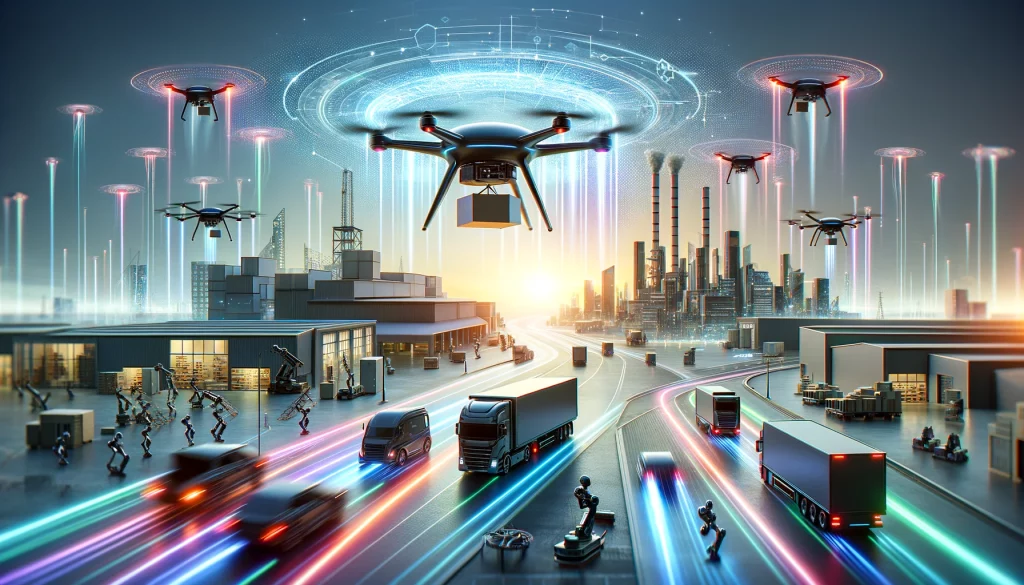
Section 3: AI in Inventory Management
3.1 The Importance of Inventory Management in Logistics
Inventory management is a critical component of supply chain operations. It involves maintaining the right level of stock in warehouses to ensure that products are available when customers need them, without overstocking or understocking. Traditional inventory management was a labor-intensive process that relied on manual checks and stock-taking, which were prone to human error.
AI has transformed inventory management by automating stock tracking, improving demand forecasting, and minimizing stockouts and overstocks.
3.2 How AI Optimizes Inventory Management
AI algorithms help businesses streamline inventory management by offering several advantages:
- Demand Forecasting: AI systems predict demand based on historical sales data, seasonal trends, and external factors like economic conditions or weather.
- Automated Replenishment: AI monitors inventory levels and automatically places orders to replenish stock based on predicted demand, reducing the risk of stockouts.
- Warehouse Optimization: AI systems help arrange products in warehouses to ensure faster picking and sorting, improving overall efficiency.
Key AI Technologies for Inventory Management:
- Predictive Analytics: AI uses past data to predict future demand, allowing companies to optimize their stock levels and avoid overstocking.
- Robotic Process Automation (RPA): Robots powered by AI can track inventory levels, move goods in warehouses, and even sort items.
- IoT Sensors and AI: AI-powered sensors in warehouses can track the real-time status of goods, allowing for precise inventory management.
3.3 Case Study: Walmart’s Inventory Optimization System
Walmart, one of the largest retailers in the world, uses AI to optimize inventory management across its vast network of stores and warehouses. By leveraging machine learning and predictive analytics, Walmart can accurately forecast demand for specific products, ensuring that the right amount of inventory is available at the right time. The system also helps the retailer reduce excess inventory and prevent stockouts.
Lessons Learned:
- The use of AI in demand forecasting improves stock levels and reduces excess inventory.
- Integrating AI with IoT sensors can significantly enhance real-time inventory visibility.
Section 4: The Future of AI in Logistics and Transportation
4.1 AI-Powered Autonomous Delivery Vehicles
Autonomous delivery vehicles, such as drones and self-driving trucks, are a major innovation in the logistics industry. AI plays a central role in the development of these technologies, enabling vehicles to navigate, avoid obstacles, and follow optimized routes without human intervention. These technologies are expected to revolutionize last-mile delivery, reducing delivery costs and improving delivery speed.
4.2 AI in Predictive Maintenance for Vehicles
AI can also be used in predictive maintenance for vehicles. By monitoring vehicle performance data, AI systems can predict when a vehicle is likely to need maintenance, preventing breakdowns and reducing downtime. This is particularly useful in logistics, where maintaining a fleet of delivery trucks is essential to ensuring smooth operations.
4.3 AI in Last-Mile Delivery
The final stage of delivery—last-mile delivery—can be the most challenging and expensive part of the supply chain. AI can improve last-mile delivery by optimizing routes, using autonomous vehicles, and enhancing package tracking systems. AI-powered systems can also manage customer preferences, such as preferred delivery times, ensuring higher customer satisfaction.
Section 5: Challenges and Ethical Considerations
While AI offers significant advantages in optimizing logistics and transportation, it also brings challenges and ethical concerns that must be addressed:
- Data Privacy and Security: AI systems require vast amounts of data, which raises concerns about data privacy and security.
- Job Displacement: Automation and AI-powered technologies could potentially replace human jobs, particularly in warehouse and driving sectors.
- High Implementation Costs: Developing and integrating AI systems into existing logistics infrastructure can be expensive for companies, especially smaller ones.
Conclusion
AI is transforming the logistics and transportation industries by optimizing route planning and inventory management, significantly improving operational efficiency and reducing costs. Through case studies like UPS’s ORION system and Walmart’s inventory optimization strategies, we can see the tangible benefits AI brings to the sector.
The future of AI in logistics is bright, with advancements in autonomous vehicles, predictive maintenance, and last-mile delivery on the horizon. However, it’s essential for businesses to consider the challenges and ethical implications of implementing AI, particularly around data privacy and workforce impacts.
As AI continues to evolve, the logistics and transportation industries are poised to become more efficient, cost-effective, and responsive to the demands of modern commerce.