Introduction
As the world becomes increasingly urbanized, cities face numerous challenges, from congestion and pollution to resource management and safety. Traditional urban planning and management methods are often insufficient to address these issues efficiently. This is where Artificial Intelligence (AI) comes into play, offering advanced solutions that enhance the livability, sustainability, and functionality of cities.
AI is revolutionizing the way cities operate by enabling more intelligent decision-making, optimizing resource allocation, and improving the quality of life for residents. From traffic management and waste collection to healthcare delivery and public safety, AI-driven technologies are integrated into various aspects of smart city development.
This article explores how AI is improving smart cities, offering practical examples and successful case studies. We will also look at how AI-powered systems can help overcome urbanization challenges and provide a roadmap for other cities looking to implement similar technologies.
Section 1: The Role of AI in Smart City Development
1.1 What is a Smart City?
A smart city uses digital technologies to enhance performance, well-being, and reduce costs & resource consumption across the city. This involves the integration of information and communication technology (ICT) to manage assets, resources, and services efficiently.
Key components of a smart city include:
- Connectivity: The infrastructure enabling data exchange between devices, systems, and people.
- Data Analytics: The process of gathering, analyzing, and using data to improve city operations and services.
- Automation: AI systems that autonomously make decisions or optimize city functions.
1.2 AI Technologies Used in Smart Cities
AI encompasses a broad range of technologies that make smart cities smarter:
- Machine Learning (ML): Machine learning algorithms analyze vast amounts of data to detect patterns and make predictions about city operations, such as predicting traffic flows, power demand, or water usage.
- Computer Vision: AI uses cameras and sensors to monitor city activities, analyze traffic, detect accidents, and track crowd movements.
- Natural Language Processing (NLP): NLP enables communication between citizens and city services through chatbots or voice-based interfaces.
- Internet of Things (IoT): IoT devices, such as sensors, cameras, and smart meters, feed real-time data to AI systems, which analyze and act on that information.
Section 2: AI-Driven Solutions in Urban Management
2.1 AI in Traffic and Transportation Management
Traffic congestion is a significant issue in most cities, leading to wasted time, fuel, and increased air pollution. AI technologies are helping cities optimize traffic flow, reduce congestion, and improve public transportation systems.
Key Applications of AI in Transportation:
- Smart Traffic Lights: AI can optimize traffic light timings in real-time based on traffic flow, reducing congestion and improving overall traffic efficiency.
- Autonomous Vehicles: AI-powered autonomous vehicles contribute to smart city infrastructure by reducing traffic congestion, enhancing road safety, and improving public transport efficiency.
- Predictive Traffic Analytics: AI algorithms analyze traffic patterns and predict potential traffic jams or accidents, helping to redirect traffic in advance and reduce delays.
Case Study: Singapore’s Smart Mobility Solutions
Singapore has been a leader in implementing AI-driven traffic management. The city-state’s smart transportation initiatives include the use of sensors and AI analytics to manage traffic flow, predict congestion, and dynamically adjust traffic signals to avoid gridlocks. The city’s Mobility-as-a-Service (MaaS) platform offers users personalized travel recommendations based on real-time data, which integrates public transport, taxis, and shared mobility services.
Lessons Learned:
- Integration of various transportation modes (public, private, shared) into one unified system.
- The importance of real-time data and predictive algorithms in traffic management.
2.2 AI in Waste Management and Sustainability
Waste management is another area where AI is making a significant impact. AI technologies optimize the collection, recycling, and disposal of waste, improving efficiency and minimizing the environmental footprint.
Key Applications of AI in Waste Management:
- Smart Waste Bins: AI-powered waste bins equipped with sensors can track waste levels and automatically signal when they need to be emptied, reducing unnecessary trips and improving resource allocation.
- AI in Recycling: AI systems are used to sort waste materials more efficiently. For instance, robots with AI can sort through recycling plants, distinguishing between different types of waste (plastic, metal, etc.) for more effective recycling.
Case Study: The City of Amsterdam’s Smart Waste Management System
Amsterdam is using AI to optimize waste management. By integrating AI-powered sensors in waste bins, the city tracks waste levels and reduces fuel consumption in garbage collection. Moreover, robots and AI-based sorting systems in recycling centers help identify materials faster, increasing recycling efficiency.
Lessons Learned:
- AI can help reduce operational costs and improve environmental sustainability.
- Integrating AI with IoT devices enhances operational efficiency and sustainability.
2.3 AI in Energy Efficiency and Smart Grids
Energy consumption is one of the largest contributors to carbon footprints in cities. Smart grids powered by AI enable more efficient management of energy resources, reduce waste, and improve energy usage patterns.
Key Applications of AI in Energy Management:
- Smart Grids: AI-driven smart grids optimize energy distribution, automatically adjusting to supply and demand changes, and enhancing overall grid stability.
- Energy Consumption Forecasting: AI algorithms analyze historical data to forecast energy consumption patterns, enabling cities to adjust energy supply in real-time and prevent blackouts.
- Renewable Energy Integration: AI helps integrate renewable energy sources, such as solar and wind, into the grid by predicting supply fluctuations and making the necessary adjustments.
Case Study: Barcelona’s Smart Grid
Barcelona has implemented a smart grid system that uses AI to predict energy demand and automatically adjust energy distribution to prevent overloads and reduce costs. AI also helps the city optimize the use of renewable energy sources by predicting fluctuations in supply from solar and wind power.
Lessons Learned:
- AI can dramatically improve the efficiency and sustainability of energy systems.
- Real-time data analysis is crucial for managing fluctuating renewable energy sources.
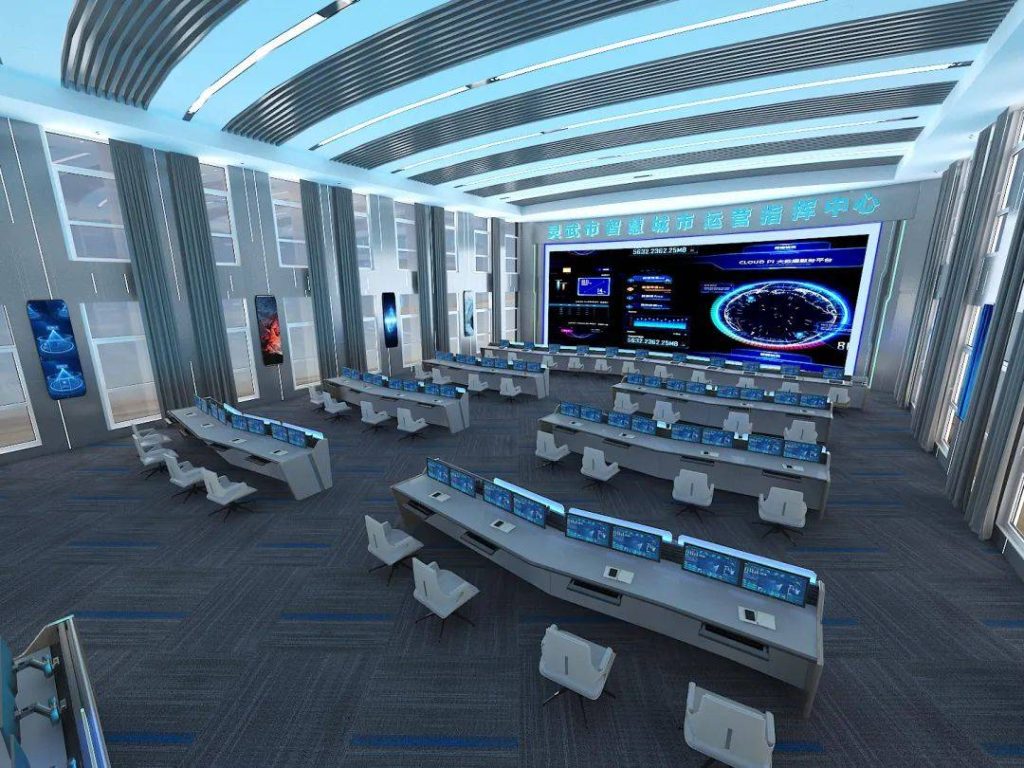
Section 3: AI in Public Safety and Emergency Response
3.1 AI for Crime Prevention and Public Security
Public safety is a core concern in any smart city. AI plays an essential role in improving surveillance, predicting crime, and enhancing emergency response systems.
Key Applications of AI in Public Safety:
- Predictive Policing: AI uses historical crime data to predict where crimes are likely to occur, enabling law enforcement to deploy resources proactively.
- AI in Surveillance: AI systems process video feeds from CCTV cameras, automatically detecting suspicious activities or identifying potential threats.
- Smart Emergency Response: AI helps optimize emergency response times by analyzing data from various sources and dispatching appropriate resources quickly.
Case Study: London’s AI-Powered Public Safety Systems
In London, AI-driven facial recognition technology and surveillance systems help track and identify criminals in crowded areas. The city also uses AI to optimize the deployment of police officers in areas predicted to have a higher risk of crime. AI helps improve response times to incidents, ensuring public safety is enhanced.
Lessons Learned:
- Combining AI with existing surveillance systems increases public security.
- Ethical concerns, particularly related to privacy, should be managed carefully when deploying AI-based surveillance.
3.2 AI in Disaster Management
In the context of natural disasters, AI is helping cities respond more quickly and efficiently. AI models predict potential risks, analyze disaster scenarios, and provide emergency response teams with actionable insights.
Key Applications of AI in Disaster Management:
- Earthquake Prediction and Early Warning: AI analyzes seismic data to detect early signs of earthquakes, providing critical warnings to residents and authorities.
- Flood and Fire Prediction: AI analyzes weather patterns, river levels, and environmental factors to predict floods or wildfires and help prevent or mitigate damage.
Case Study: AI-Driven Flood Prediction in India
In India, the National Disaster Management Authority has implemented AI to predict flood risks in real-time. AI algorithms analyze weather forecasts, river conditions, and satellite data to provide early warnings to communities, helping them evacuate and prepare ahead of time.
Lessons Learned:
- AI can significantly improve disaster preparedness and response times.
- Collaborating with local governments and communities is crucial for effective implementation.
Section 4: Challenges and Ethical Considerations
While AI offers tremendous benefits for smart cities, there are several challenges that need to be addressed:
- Privacy Concerns: AI-powered surveillance systems and data collection may infringe upon individuals’ privacy if not managed properly.
- Data Security: As AI systems collect and analyze vast amounts of data, safeguarding this information against cyber threats is critical.
- Bias in AI: AI systems can perpetuate biases in decision-making, especially in predictive policing and hiring algorithms.
- High Implementation Costs: Developing and deploying AI infrastructure in a smart city can be costly, requiring significant investment from local governments and stakeholders.
Conclusion
Artificial intelligence is playing a pivotal role in the development of smart cities, improving everything from traffic management and waste collection to public safety and emergency response. Through various applications, AI is helping cities optimize resources, improve efficiency, and enhance the quality of life for residents.
The case studies of cities like Singapore, Barcelona, and London showcase the potential of AI in smart city development, offering valuable lessons for other urban areas looking to leverage these technologies. However, successful implementation requires careful planning, addressing ethical concerns, and ensuring that AI is deployed in ways that are transparent and beneficial to all citizens.
The future of smart cities lies in the seamless integration of AI technologies that work together to create sustainable, efficient, and livable urban spaces for generations to come.