Introduction
In today’s rapidly evolving technological landscape, AI (Artificial Intelligence) is becoming a cornerstone of many industries, driving innovation, efficiency, and new business opportunities. As organizations adopt AI solutions to transform operations, it’s essential to assess and choose the right AI resources to ensure that projects are successfully implemented.
Evaluating and selecting AI resources involves considering various factors, including the complexity of the project, the specific requirements of the organization, budget constraints, and the desired outcomes. The wrong choices in resources can lead to project delays, cost overruns, and unsatisfactory results. This article aims to provide detailed guidelines on how to effectively evaluate and choose the most suitable AI resources to maximize the chances of successful project execution.
1. Understanding the AI Project Requirements
1.1 Defining the Problem and Objectives
Before selecting any AI tools or resources, it is critical to define the problem the AI project is aiming to solve. This involves:
- Clarifying business goals and objectives
- Identifying the scope of the AI implementation (e.g., automation, predictive modeling, data analysis, customer service enhancement)
- Understanding key performance indicators (KPIs) that will define success
Example: An AI-powered customer service chatbot will have different requirements compared to a deep learning model for medical image analysis.
1.2 Assessing Data Requirements
Data is at the core of any AI project. Analyzing the type, volume, and quality of the data you have or can collect is key:
- Is the data structured or unstructured?
- Do you have sufficient data for training the AI models?
- Are there any data privacy or regulatory considerations?
The availability and quality of data will heavily influence the choice of tools and platforms for model training, deployment, and maintenance.
2. Evaluating AI Platforms and Tools
2.1 Types of AI Resources
There are various AI resources available, ranging from open-source libraries to enterprise-level platforms. These resources can be categorized into the following:
- AI Development Frameworks (e.g., TensorFlow, PyTorch): These are libraries that provide developers with tools for building and training machine learning models.
- Cloud-Based AI Platforms (e.g., AWS AI, Google Cloud AI, Microsoft Azure): These platforms provide ready-to-use AI models, as well as infrastructure to train and deploy custom AI applications.
- AI as a Service (e.g., IBM Watson, OpenAI API): Pre-built AI solutions such as chatbots, language translation, and image recognition, available through APIs.
- No-Code/Low-Code AI Tools (e.g., Teachable Machine, Runway ML): Tools that allow non-technical users to build AI models with little to no coding experience.
2.2 Key Features to Look for in AI Resources
When evaluating AI tools and platforms, it’s important to consider the following features:
- Scalability: Can the tool handle growing data and usage over time?
- Ease of Use: Does the tool offer a user-friendly interface or require advanced technical expertise?
- Integration Capabilities: Can the tool easily integrate with existing systems and platforms within the organization (e.g., CRM, ERP)?
- Performance and Speed: Does the platform provide the necessary computational power and speed to run AI models efficiently?
- Security and Compliance: Does the platform comply with data protection laws and offer adequate security measures for sensitive data?
2.3 Open-Source vs. Proprietary Tools
Choosing between open-source AI tools and proprietary platforms is a critical decision:
- Open-Source Tools: These tools are free to use and customizable, with strong community support. However, they require expertise to integrate and maintain.
- Proprietary Tools: These solutions are typically user-friendly and come with vendor support but may involve licensing fees.
The choice between open-source and proprietary tools depends on your organization’s technical capabilities, budget, and long-term goals.
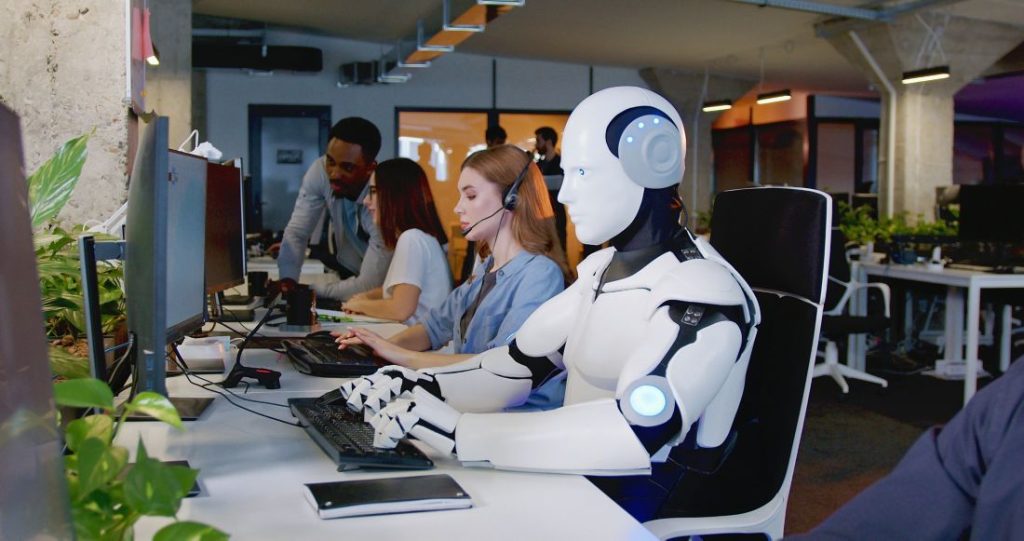
3. Assessing Skillsets and Expertise Required
3.1 Understanding the Team’s Capabilities
AI projects require specialized skills in areas such as:
- Data Science and Machine Learning: Knowledge of algorithms, model training, and evaluation.
- Software Development: Expertise in integrating AI models into existing systems.
- Cloud and Infrastructure: Understanding of cloud platforms and infrastructure to support AI applications.
- Business Acumen: The ability to translate AI insights into actionable business strategies.
Assessing the current skillset of your team will help determine whether you need to hire specialists, invest in training, or choose tools that minimize the need for advanced technical expertise.
3.2 Training and Support Needs
For teams with limited AI experience, it’s crucial to evaluate the training resources, tutorials, and support provided by the AI tool or platform. Some platforms offer extensive learning materials, certifications, and community forums to assist beginners, while others may require more advanced knowledge to utilize effectively.
4. Budgeting and Cost Considerations
4.1 Initial Investment vs. Long-Term Costs
The cost of AI resources can vary significantly depending on the platform, the complexity of the project, and the scale at which it will operate. It’s important to assess:
- Upfront costs: Subscription fees, hardware, training costs
- Ongoing costs: Cloud compute expenses, API usage, maintenance, and updates
Example: While open-source tools may be free to use, the cost of implementing and maintaining them could be higher in terms of staff time and infrastructure requirements.
4.2 Return on Investment (ROI)
Consider the potential return on investment from implementing AI solutions. AI can significantly improve productivity, reduce costs, and enhance decision-making, but the financial impact of AI implementation should be assessed based on:
- Efficiency gains
- Cost savings
- Revenue growth
Calculating ROI helps determine whether the investment in AI tools and resources is justified.
5. Evaluating Vendors and Service Providers
5.1 Vendor Reputation and Experience
When selecting a vendor or service provider, consider the following:
- Track Record: Does the vendor have experience delivering successful AI solutions in your industry?
- Client Testimonials: What do previous clients say about their experience with the vendor’s AI tools?
- Product Longevity: Will the vendor continue to develop and support the platform in the long term?
Evaluating the vendor’s reputation, product roadmap, and commitment to innovation is crucial to ensure your project’s long-term success.
5.2 Customization and Flexibility
It’s essential to determine whether the AI tool or platform can be customized to meet the unique needs of your organization. Some vendors provide flexible solutions that can be tailored to specific use cases, while others may offer more rigid, out-of-the-box solutions.
5.3 Customer Support and Service Level Agreements (SLAs)
AI projects require continuous support, especially when challenges arise during deployment or maintenance. Ensure that the vendor provides:
- Responsive customer support
- Clear SLAs detailing the expected response times and resolution procedures
- Ongoing maintenance and upgrades
6. Ensuring Ethical Considerations and Regulatory Compliance
6.1 Data Privacy and Ethics
AI projects often involve handling sensitive data, making it critical to ensure compliance with data protection laws such as GDPR, CCPA, or HIPAA. Choose AI platforms and tools that adhere to ethical standards and prioritize user privacy.
6.2 Bias and Fairness in AI
AI models can sometimes inherit biases from training data, leading to unfair or discriminatory outcomes. Evaluate the AI resource’s ability to:
- Mitigate bias in the models
- Provide transparency and explainability
- Ensure fairness in AI-driven decisions
Ethical considerations should be at the forefront when selecting AI tools for any project.
7. Testing and Piloting AI Resources
7.1 Running a Pilot Project
Before fully committing to a particular AI resource, it’s often beneficial to run a pilot project. This allows the team to assess the tool’s effectiveness, scalability, and compatibility with the organization’s existing infrastructure.
7.2 Monitoring Performance
Once the pilot project is completed, monitor key metrics to evaluate the success of the implementation. Key performance indicators (KPIs) might include:
- Model accuracy
- Cost savings
- Time savings
- User adoption rates
This data will help inform the decision to scale up or make adjustments.
8. Continuous Improvement and Adaptation
AI is a rapidly evolving field, and it’s essential to stay updated with the latest tools and trends. As your AI project grows, it’s important to continually assess and adapt your resources to keep up with new technologies and methodologies.
Conclusion
Choosing the right AI resources is a critical step in ensuring the success of your project. By thoroughly evaluating your organization’s needs, selecting the appropriate tools, assessing vendor capabilities, and considering ethical implications, you can significantly increase the likelihood of achieving successful outcomes. Remember, the selection process is not a one-time task but an ongoing effort that requires flexibility and a commitment to continuous learning and adaptation.
By following the steps outlined in this article, organizations can make informed decisions, streamline AI adoption, and drive long-term success in AI initiatives.