Deep learning has emerged as one of the most transformative forces in artificial intelligence (AI) in recent years, and it has undoubtedly played a major role in pushing the boundaries of what AI can achieve. But is deep learning the key to AI’s future? And how is it shaping the next generation of AI models?
This article explores deep learning’s impact on the AI landscape, its application across industries, and the potential of emerging models that could revolutionize the field. From natural language processing (NLP) to computer vision, deep learning has enabled AI to perform complex tasks that were previously considered unattainable. But as the AI field continues to evolve, we also look at the challenges and limitations of deep learning and consider what the future holds for AI models.
What is Deep Learning?
Deep learning, a subfield of machine learning, is centered around artificial neural networks, which are designed to simulate the way human brains process information. These neural networks consist of layers of interconnected nodes (or neurons) that process data through a series of computations. Deep learning algorithms are designed to automatically learn patterns and features in data by training on large datasets. Unlike traditional machine learning algorithms, deep learning models can learn directly from raw data, such as images, text, and audio, without the need for manual feature extraction.
At the core of deep learning are neural networks, particularly deep neural networks (DNNs), which consist of multiple layers (hence the term “deep”) of neurons that allow the model to learn hierarchical features in the data. These networks can automatically extract low-level features in the initial layers and then combine them into more complex and abstract features in higher layers, enabling deep learning models to make predictions, classifications, and decisions with remarkable accuracy.
The Evolution of Deep Learning Models
Deep learning has its roots in neural networks that date back to the 1950s, but it wasn’t until the 2000s, with the availability of large datasets and powerful computational resources, that deep learning really began to take off. The success of deep learning can be attributed to several key factors:
- Data Availability: The rise of big data and the availability of vast datasets from various sources, such as social media, e-commerce, healthcare, and autonomous vehicles, has provided the fuel for deep learning models. The larger the dataset, the more accurate and capable the deep learning model becomes.
- Computational Power: The availability of high-performance computing, particularly Graphics Processing Units (GPUs), has enabled researchers to train deep learning models much faster than ever before. The ability to process large datasets quickly and efficiently has accelerated the development of deep learning algorithms.
- Algorithmic Advances: Over the years, deep learning researchers have developed new algorithms, such as convolutional neural networks (CNNs), recurrent neural networks (RNNs), and transformers, each designed for specific tasks like image recognition, language modeling, and sequential data processing. These innovations have allowed deep learning to achieve state-of-the-art performance in a variety of applications.
Deep Learning’s Impact on AI Applications
Deep learning has significantly advanced the capabilities of AI across a wide range of applications. Below are some of the most impactful areas where deep learning has made significant strides:
1. Natural Language Processing (NLP)
Deep learning has revolutionized the field of natural language processing, enabling machines to understand and generate human language at a level of sophistication never seen before. Techniques such as transformers and attention mechanisms have paved the way for models like OpenAI’s GPT-3 and Google’s BERT, which can generate coherent text, translate languages, summarize content, and even answer questions with human-like accuracy.
In recent years, deep learning-based NLP models have enabled significant improvements in sentiment analysis, chatbots, virtual assistants, and automated content generation. These models use vast amounts of text data to learn grammar, syntax, and meaning, enabling them to handle tasks ranging from text classification to machine translation.
2. Computer Vision
Deep learning has also revolutionized computer vision, allowing AI to recognize, interpret, and generate visual data with exceptional precision. Convolutional neural networks (CNNs), a type of deep learning model, are at the core of many computer vision tasks, including image classification, object detection, facial recognition, and image segmentation.
CNNs have been used to achieve human-level performance in a variety of computer vision benchmarks, including the ImageNet competition, where deep learning models have consistently outperformed traditional computer vision techniques. Applications of deep learning in computer vision are widespread, from self-driving cars and surveillance systems to medical imaging and content moderation.
3. Autonomous Vehicles
The development of autonomous vehicles relies heavily on deep learning models that enable self-driving cars to navigate complex environments. These models use data from sensors such as cameras, lidar, and radar to process and interpret the car’s surroundings. Deep learning algorithms are responsible for object detection, path planning, and decision-making in autonomous driving systems.
Deep learning allows AVs to detect pedestrians, other vehicles, and road signs, as well as make split-second decisions in dynamic environments. By continually improving their ability to perceive and react to changes in the environment, deep learning models are paving the way for the widespread adoption of autonomous vehicles.
4. Healthcare and Medicine
Deep learning has demonstrated immense potential in healthcare, particularly in areas like medical imaging, drug discovery, and personalized treatment. AI models trained on medical data such as X-rays, MRIs, and CT scans have been able to assist doctors in diagnosing diseases like cancer, Alzheimer’s, and cardiovascular conditions.
For example, deep learning models have been trained to identify early signs of diseases in medical images, providing doctors with a powerful tool for early detection and improving patient outcomes. Additionally, deep learning is being used in drug discovery to predict the properties of new molecules and identify potential candidates for new treatments.
5. Robotics
Deep learning is also making waves in robotics, where it is enabling robots to perform complex tasks that were once reserved for humans. From warehouse automation to surgical robots, deep learning algorithms allow robots to interact with their environment, process sensory input, and adapt to changing conditions in real time.
Robots powered by deep learning models can perform tasks such as object manipulation, assembly line work, and even assist with surgery by providing real-time feedback to surgeons. These advances in robotics are helping to reduce labor costs and improve efficiency in a wide range of industries.
Challenges and Limitations of Deep Learning
Despite its remarkable successes, deep learning is not without its challenges. As deep learning models become increasingly complex, they require vast amounts of data, computational power, and time to train. Here are some of the main limitations of deep learning:
1. Data Dependency
Deep learning models are highly dependent on large, high-quality datasets to perform well. Without sufficient data, models can overfit or underperform, leading to suboptimal results. In some fields, such as healthcare or law, obtaining large datasets can be difficult due to privacy concerns or the cost of data collection.
2. Interpretability and Explainability
One of the biggest challenges with deep learning models is their lack of interpretability. These models are often considered “black boxes” because it’s difficult to understand how they arrive at certain decisions. In fields like healthcare and finance, where transparency is crucial, the inability to explain how a model works can be a significant barrier to adoption.
3. Computational Costs
Training deep learning models can be computationally expensive, requiring powerful hardware like GPUs or specialized processors. The energy consumption required to train large models can also have a significant environmental impact, raising concerns about the sustainability of deep learning research.
4. Generalization Issues
Deep learning models tend to perform well on tasks they have been specifically trained on but can struggle when faced with new or unseen data. This lack of generalization can limit the applicability of deep learning in certain real-world scenarios, where conditions can change rapidly and unpredictably.
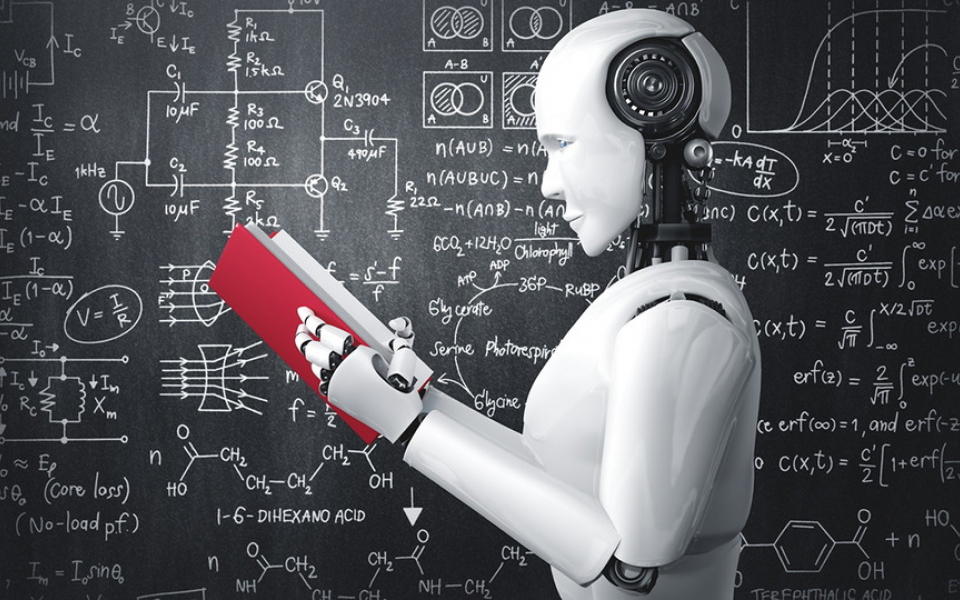
The Future of Deep Learning: What’s Next?
The future of deep learning looks bright, with several exciting trends and developments on the horizon. As AI researchers continue to refine deep learning models and overcome their limitations, we can expect to see even more groundbreaking advancements in the coming years. Some of the most promising areas of deep learning research include:
1. Few-Shot and Zero-Shot Learning
Few-shot learning, and its more advanced cousin, zero-shot learning, aim to enable AI systems to learn new tasks with very little data. These techniques allow models to generalize to new scenarios without requiring large amounts of training data, making deep learning more flexible and applicable to a wider range of applications.
2. Self-Supervised Learning
Self-supervised learning is an emerging paradigm that aims to reduce the reliance on labeled data. In self-supervised learning, the model learns to generate its own labels from the input data, effectively creating a label-free training process. This could dramatically reduce the amount of manual effort required to prepare data for training, making deep learning more accessible and scalable.
3. Ethical AI and Fairness
As deep learning becomes more widespread, addressing ethical concerns and ensuring fairness in AI systems will become increasingly important. Researchers are actively working on developing methods to ensure that deep learning models do not perpetuate biases or make unfair decisions, particularly in sensitive areas like criminal justice, hiring, and lending.
4. Quantum Deep Learning
Quantum computing has the potential to significantly accelerate deep learning by allowing models to process vast amounts of data much faster than classical computers. Researchers are exploring how quantum computing can be integrated with deep learning models to enhance their capabilities and performance, potentially unlocking new frontiers in AI research.
Conclusion: The Road Ahead for Deep Learning
Deep learning has already had a profound impact on AI, driving advances in fields like natural language processing, computer vision, healthcare, and robotics. As we look to the future, deep learning will continue to push the boundaries of what is possible in AI. However, challenges such as data dependency, interpretability, and computational costs must be addressed to unlock the full potential of deep learning.
With ongoing advancements in AI research, emerging techniques like few-shot learning, self-supervised learning, and quantum deep learning, deep learning is poised to play a central role in shaping the future of AI. As the next generation of AI models evolves, deep learning will remain at the heart of the revolution, helping to solve some of the world’s most pressing problems and unlock new opportunities for innovation across industries.
Article Tags
Deep learning, AI models, machine learning, neural networks, artificial intelligence, natural language processing, computer vision, autonomous vehicles, robotics, healthcare AI, deep learning applications, AI advancements, AI challenges, future of AI, quantum computing and AI, ethical AI, AI research.
Image Search Keywords
deep learning, AI models, neural networks, AI in healthcare, computer vision AI, autonomous vehicles, robotics AI, natural language processing, machine learning, AI research.