Quantum computing, once the stuff of science fiction, is now a rapidly evolving field that promises to revolutionize industries ranging from finance to healthcare. Its potential to drastically enhance artificial intelligence (AI) capabilities is one of the most exciting areas of research today. With the help of quantum mechanics, quantum computers have the ability to process vast amounts of information far more efficiently than traditional computers, offering unprecedented opportunities to advance machine learning, data analysis, and decision-making processes.
This article explores how quantum computing is being used to enhance AI capabilities, the latest trends, breakthroughs, and the challenges that come with this intersection of two cutting-edge fields. We will examine the potential applications of quantum AI, the progress made by tech giants and researchers, and what the future may hold for the synergy between these two technologies.
Understanding Quantum Computing: The Basics
Before diving into the specifics of how quantum computing enhances AI, it’s essential to understand what quantum computing is and why it’s so different from classical computing. Traditional computers, like the one you’re reading this article on, process data in binary form, meaning information is represented by bits that can either be a 0 or a 1. These bits are processed using conventional algorithms and logic gates.
Quantum computers, however, operate based on principles of quantum mechanics, which govern the behavior of particles at a microscopic scale. Quantum bits, or qubits, are the fundamental units of quantum computing. Unlike classical bits, qubits can exist in multiple states simultaneously due to a property called superposition. This allows quantum computers to process and solve complex problems in parallel, offering an exponential speed-up in computations for certain tasks.
Another crucial concept in quantum computing is entanglement, where the state of one qubit is dependent on the state of another, no matter how far apart they are. This allows quantum computers to solve problems with greater efficiency, particularly when dealing with tasks that involve large amounts of data or require advanced calculations.
Quantum Computing and AI: The Perfect Pairing
Artificial intelligence is inherently data-driven, and the ability to process massive datasets efficiently is central to AI’s success. Traditional computing has limitations when it comes to solving particularly complex or computationally expensive tasks. This is where quantum computing’s parallel processing power can significantly enhance AI capabilities.
The following sections outline several ways in which quantum computing is enhancing AI.
1. Speeding Up Machine Learning Algorithms
Machine learning, a subset of AI, relies on algorithms that learn from data to make predictions or decisions. The training process for these algorithms often involves running computations on large datasets, which can be very time-consuming on classical computers. Quantum computing can accelerate this process by enabling faster matrix multiplications, a key operation in machine learning.
Quantum computers can handle operations in superposition, processing many possibilities at once, as opposed to classical computers, which process them sequentially. This means that tasks such as gradient descent, which is used to minimize errors in machine learning models, could potentially be accelerated through quantum algorithms, significantly speeding up the training phase of AI models.
Quantum-enhanced machine learning (QML) can also reduce the computational cost of analyzing and interpreting large datasets. Quantum algorithms, such as quantum support vector machines and quantum neural networks, show promise in efficiently classifying and clustering data, which can be incredibly useful for applications like image recognition, natural language processing (NLP), and financial forecasting.
2. Optimization Problems: A Quantum Leap
Optimization is a critical aspect of AI, particularly in areas such as logistics, supply chain management, and recommendation systems. Optimization problems involve finding the best solution from a set of possible choices, and they are often computationally intensive. Classical computers can struggle with large-scale optimization problems due to their limited processing power.
Quantum computers have the potential to solve optimization problems more efficiently through quantum algorithms such as quantum annealing and the quantum approximate optimization algorithm (QAOA). These algorithms use quantum mechanics to explore large solution spaces and converge on optimal or near-optimal solutions more quickly than classical algorithms.
For example, D-Wave Systems, a company at the forefront of quantum computing, has developed quantum annealing technology that can be applied to optimization problems. AI applications in logistics, scheduling, and planning can significantly benefit from these advancements, providing faster and more accurate solutions to complex optimization challenges.
3. Quantum AI in Drug Discovery and Healthcare
The healthcare industry stands to benefit tremendously from the combination of AI and quantum computing. Drug discovery, for example, is a notoriously slow and expensive process, with researchers needing to analyze vast amounts of chemical data to identify potential compounds. Quantum computers can accelerate this process by simulating molecular interactions more efficiently than classical computers, leading to faster drug discovery and more effective treatments.
AI plays a pivotal role in predicting the behavior of molecules, optimizing drug compounds, and analyzing clinical trial data. Quantum computing enhances this by providing the computational power needed to simulate and analyze large molecular structures, improving AI’s ability to make predictions about chemical interactions and biological processes.
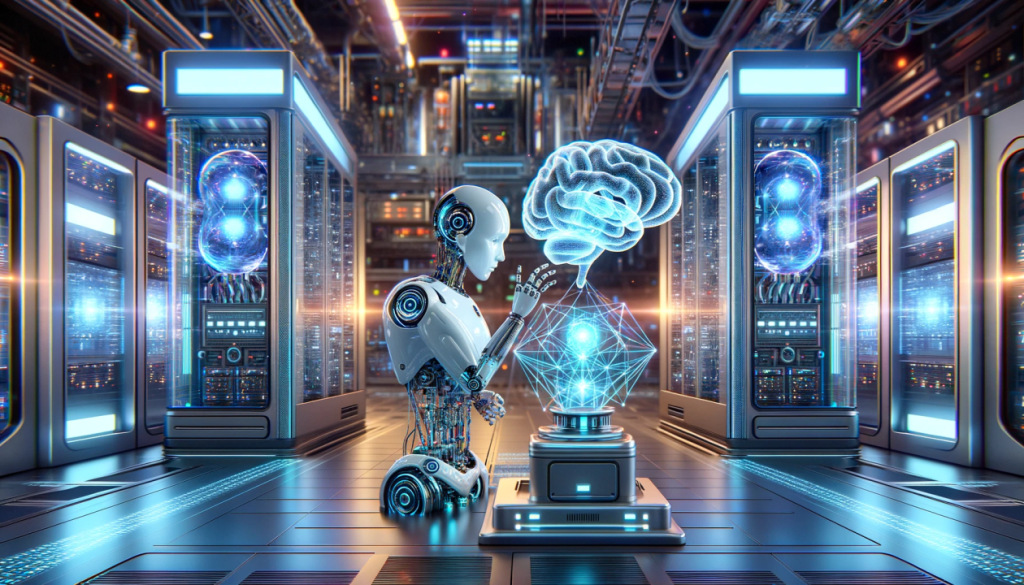
4. Quantum-enhanced Natural Language Processing (NLP)
Natural Language Processing (NLP) is another area of AI that stands to benefit from quantum computing. NLP involves teaching machines to understand, interpret, and generate human language, which is a highly complex task due to the vast nuances of language.
Quantum computing’s ability to process vast amounts of data simultaneously can greatly enhance NLP tasks such as language translation, sentiment analysis, and speech recognition. Quantum algorithms can help AI models process language patterns and relationships more efficiently, leading to more accurate and nuanced language understanding. Quantum models may also be able to handle larger vocabularies and more complex linguistic structures, further enhancing the capabilities of NLP systems.
5. Quantum Machine Learning in Financial Services
The financial services industry is another sector poised to benefit from quantum computing’s integration with AI. In areas such as risk assessment, fraud detection, and portfolio optimization, AI and quantum computing can offer substantial improvements over traditional methods.
Quantum-enhanced machine learning can be used to analyze large financial datasets and generate more accurate predictions about market behavior. Quantum algorithms have the potential to solve complex financial optimization problems, such as minimizing portfolio risk while maximizing returns, faster than classical algorithms. This can lead to more informed decision-making, reduced risk, and improved investment strategies.
Challenges of Integrating Quantum Computing with AI
While the potential of quantum computing to enhance AI capabilities is immense, there are still significant challenges in integrating these two technologies. Some of the major hurdles include:
- Quantum Hardware Limitations: Quantum computers are still in the early stages of development, with many companies working on building scalable quantum processors. Current quantum hardware is prone to noise and errors, which can affect the accuracy of computations.
- Algorithm Development: While quantum algorithms are being developed, there is still a lack of mature quantum machine learning algorithms that can be easily applied to real-world AI tasks. Researchers are working to develop hybrid quantum-classical approaches that combine the strengths of both types of computing.
- Scalability: Quantum computers require highly controlled environments, including ultra-low temperatures, which makes them difficult to scale for widespread use. Advances in quantum hardware are needed before these systems can become widely accessible to AI researchers and developers.
Industry Leaders and Quantum AI Research
Several tech giants are actively pursuing the intersection of quantum computing and AI. For example:
- IBM: IBM has been at the forefront of quantum computing research with its IBM Quantum platform. The company has made significant strides in developing quantum algorithms for machine learning and optimization. IBM’s Qiskit software framework is widely used by researchers to develop and test quantum algorithms for AI applications.
- Google: Google is another leader in quantum computing, with its Quantum AI division working on quantum-enhanced machine learning. In 2019, Google achieved quantum supremacy with its 53-qubit quantum computer, Sycamore, demonstrating its ability to solve a problem that would be infeasible for classical computers.
- Microsoft: Microsoft’s Azure Quantum platform is working on building quantum computing solutions for various industries, including AI. The company is focused on developing quantum algorithms and hardware that will enable AI advancements in areas such as drug discovery and material science.
- D-Wave: D-Wave offers quantum computing solutions specifically designed for optimization problems. The company’s Quantum Annealing platform has been used in AI applications for optimization in areas like supply chain management, finance, and logistics.
The Future of Quantum Computing and AI
The future of quantum computing and AI is full of promise, but it will require continued innovation and research. As quantum hardware improves and quantum algorithms are refined, AI applications across industries such as healthcare, finance, logistics, and natural language processing will benefit from more efficient computations, faster decision-making, and more accurate predictions.
In the coming years, we can expect the emergence of new hybrid models that combine quantum computing’s power with classical AI techniques. These hybrid systems will leverage the strengths of both technologies, enabling businesses and researchers to tackle problems that were previously considered intractable.
Conclusion: Quantum AI – The Future of Intelligent Systems
Quantum computing has the potential to revolutionize artificial intelligence by providing the computational power necessary to tackle some of the most complex challenges of the modern world. From accelerating machine learning algorithms to optimizing financial portfolios, the synergy between quantum computing and AI opens up a wealth of possibilities. However, realizing this potential will require overcoming significant technical challenges, including improving quantum hardware, developing new quantum algorithms, and ensuring the scalability of quantum systems. As the field progresses, we may soon see AI applications powered by quantum computing that far exceed the capabilities of today’s traditional systems.