Introduction:
In the digital age, big data has become one of the most influential tools for companies looking to enhance their decision-making capabilities. With the vast amounts of data generated every day, businesses are increasingly relying on big data analytics to not only make informed decisions but also to anticipate future trends, streamline operations, and gain a competitive edge. As a result, big data has revolutionized how companies optimize their strategies and operational processes.
Leading companies from various industries have successfully harnessed the power of big data to improve decision-making in real-time, reduce costs, increase efficiency, and deliver more personalized products and services. This article delves into how these companies are leveraging big data analytics to optimize their decision-making processes, focusing on specific examples, strategies, and the impact on business performance.
1. Data-Driven Decision-Making: The Core of Modern Business Strategy
Big data analytics involves processing large volumes of structured and unstructured data to uncover hidden patterns, correlations, trends, and insights. By analyzing data in real-time or near-real-time, businesses can make more accurate, data-driven decisions. Predictive analytics, machine learning, and artificial intelligence (AI) are key technologies that drive these processes, helping companies move beyond intuition-based decision-making to evidence-based strategies.
1.1. Transforming Raw Data into Actionable Insights
Leading companies often generate a substantial amount of raw data through customer interactions, supply chain transactions, social media, and IoT (Internet of Things) sensors. However, the challenge lies in transforming this overwhelming volume of data into actionable insights that can drive better business decisions. By using advanced analytics tools, companies are able to sort, analyze, and interpret data quickly, making it easier to extract meaningful insights.
For example, companies like Amazon and Netflix utilize big data analytics to track customer preferences, purchase behavior, and viewing patterns, allowing them to offer personalized recommendations. Similarly, Tesla uses data from its connected vehicles to enhance the performance of its cars and optimize the driving experience for users.
1.2. Predictive Analytics for Informed Decisions
One of the most significant advantages of big data analytics is the ability to leverage predictive analytics to forecast future trends and behavior. This empowers businesses to take a proactive approach rather than a reactive one. Predictive models can analyze past data to predict customer behavior, sales trends, demand forecasting, and market conditions.
Companies such as Walmart and Target use predictive analytics to optimize inventory management, predict stock levels, and anticipate demand surges, especially during peak shopping seasons. By having a better understanding of consumer behavior, they can make data-driven decisions to ensure that they have the right products in the right locations at the right time.
2. Leading Companies Leveraging Big Data for Optimized Decision-Making
Let’s take a closer look at how some of the world’s leading companies are utilizing big data analytics to optimize their decision-making processes:
2.1. Amazon: Enhancing Supply Chain and Personalization
Amazon, the world’s largest e-commerce retailer, has fully embraced big data analytics to optimize its decision-making, particularly in supply chain management and personalized customer experiences.
2.1.1. Optimizing Inventory Management
With millions of products being sold globally, Amazon relies heavily on big data analytics to manage its vast inventory across warehouses and distribution centers. By analyzing historical sales data, customer preferences, and market trends, Amazon predicts demand for specific products at specific locations, allowing them to optimize their inventory distribution and reduce stockouts or excess inventory.
For instance, Amazon’s algorithm analyzes purchasing patterns in real time to adjust the inventory levels in warehouses, ensuring that products are available for quick shipping to customers. By streamlining its supply chain using big data, Amazon reduces operating costs and increases the efficiency of its deliveries, which is a major factor in its success.
2.1.2. Personalizing Customer Experience
Amazon also uses big data analytics to personalize the shopping experience for each customer. By analyzing data on previous purchases, browsing history, and search behavior, Amazon delivers tailored recommendations to customers. This not only enhances the shopping experience but also increases conversion rates and customer retention.
2.2. Netflix: Data-Driven Content Strategy
As a leader in the streaming industry, Netflix has leveraged big data to revolutionize its content strategy, improving user engagement and retention. The company analyzes vast amounts of data on user preferences, viewing habits, and social media activity to make better decisions about what content to produce or license.
2.2.1. Personalized Content Recommendations
Netflix’s personalized recommendation engine is one of the most successful applications of big data analytics in the entertainment industry. The platform analyzes data from millions of users to identify patterns and preferences, enabling it to suggest shows and movies that align with individual tastes. This has become a key factor in keeping users engaged with the platform, as viewers are more likely to stay subscribed when they’re presented with content that matches their interests.
2.2.2. Content Production Decisions
Netflix also uses big data analytics to inform decisions about which shows and movies to produce. By analyzing data on viewer engagement, completion rates, and genre preferences, Netflix can decide which content is likely to be successful. For example, the production of “House of Cards” was partly driven by the data insights Netflix gathered from user behavior, which indicated a strong interest in political dramas.
2.3. Uber: Optimizing Ride-Hailing and Dynamic Pricing
Uber is another company that has harnessed the power of big data to optimize decision-making in real-time. With millions of rides occurring daily across the world, Uber uses big data analytics to manage its driver network, optimize pricing, and enhance the overall user experience.
2.3.1. Dynamic Pricing and Demand Forecasting
Uber uses dynamic pricing, also known as surge pricing, which is driven by real-time data analytics. By analyzing data such as traffic conditions, weather, time of day, and the number of available drivers, Uber can adjust fares based on demand in a specific location. This not only ensures that drivers are incentivized to work during peak times but also helps to balance supply and demand, resulting in quicker ride availability for passengers.
2.3.2. Route Optimization
Uber uses big data analytics to optimize routes for drivers, minimizing wait times and improving fuel efficiency. The company’s algorithms process traffic data, construction zone updates, accidents, and other variables to suggest the best routes for drivers to take, ensuring that passengers reach their destinations as quickly as possible.
2.4. Target: Enhancing Customer Loyalty and Inventory Management
Retail giant Target has effectively employed big data analytics to optimize inventory management and enhance customer loyalty.
2.4.1. Personalized Marketing and Targeted Promotions
Target uses big data to analyze customer purchasing habits, demographic data, and even online browsing behaviors to send highly personalized promotions and offers. This targeted approach increases the likelihood of customer engagement and conversion. For instance, if a customer frequently purchases baby products, Target might send them coupons for diapers or baby food, increasing the chances of repeat purchases.
2.4.2. Optimizing Inventory Levels
Like other major retailers, Target relies on big data analytics for demand forecasting and inventory management. By analyzing historical sales data, market trends, and even local events, Target can predict which products will be in high demand in different locations. This helps reduce overstock or understock situations and ensures that customers find the products they want in stock.
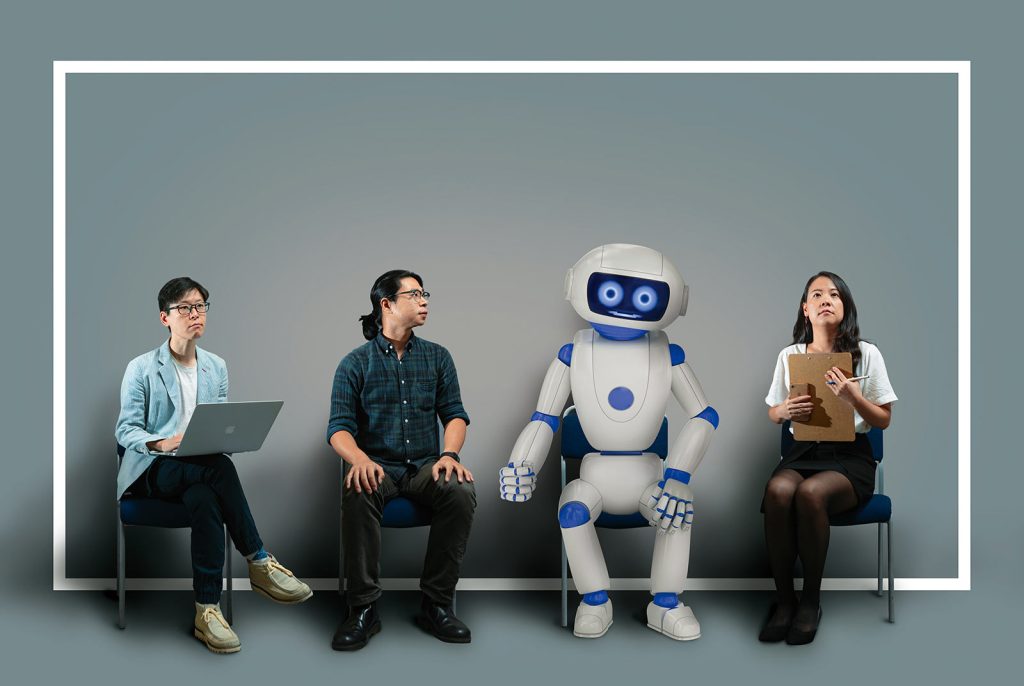
3. Challenges in Implementing Big Data Analytics
Despite the clear advantages of big data analytics, leading companies face several challenges in its implementation. These challenges must be addressed for companies to fully optimize their decision-making processes:
3.1. Data Privacy and Security Concerns
With large amounts of data being collected, companies must ensure that they comply with data protection regulations such as GDPR and CCPA. Ensuring that customer data is secure and used responsibly is critical in maintaining trust.
3.2. Data Quality and Integration
Companies need to ensure that the data they collect is accurate, complete, and consistent. Integrating data from various sources, such as internal systems, third-party platforms, and customer interactions, can be complex and require significant resources.
3.3. Skilled Talent and Technological Infrastructure
To fully leverage big data, companies need to invest in skilled data scientists, analysts, and engineers who can interpret complex data sets and develop actionable insights. Additionally, the right technological infrastructure, such as cloud computing platforms and big data processing tools, is essential for efficient data storage, analysis, and visualization.
Conclusion: The Future of Big Data in Decision-Making
As more companies recognize the value of big data analytics, the role of data-driven decision-making will continue to expand. From Amazon’s supply chain optimization to Netflix’s content strategy and Uber’s dynamic pricing, big data has proven to be a game-changer for businesses across industries.
The future of big data in decision-making will likely involve even more advanced applications of artificial intelligence, machine learning, and real-time analytics, allowing businesses to make decisions faster, more accurately, and more efficiently. However, companies must also address the challenges associated with data privacy, integration, and the need for skilled talent to fully unlock the potential of big data.
In the end, businesses that effectively leverage big data analytics will be better positioned to gain a competitive advantage, drive innovation, and ultimately achieve long-term success in an increasingly data-driven world.