Introduction
In today’s rapidly evolving digital landscape, businesses are increasingly looking to Artificial Intelligence (AI) to enhance operations, drive innovation, and improve customer experiences. However, choosing the right AI tools that align with a company’s existing technology stack is crucial for ensuring smooth integration, scalability, and long-term success.
A “technology stack” refers to the combination of software and hardware technologies that a company uses to build and maintain its applications, platforms, and services. Whether the business is using cloud services, databases, operating systems, or APIs, the compatibility of AI tools with the existing technology stack can significantly impact the implementation process.
This article explores how businesses can evaluate AI tools with a focus on their compatibility with existing technologies, the steps required to ensure seamless integration, and practical insights from real-world case studies.
Section 1: Understanding the Importance of Compatibility
1.1 Defining Compatibility in the Context of AI Tools
Compatibility, in this context, refers to the ability of AI tools to work harmoniously with a company’s existing systems, applications, and infrastructure. It includes:
- Software Compatibility: Ensuring that the AI tools can integrate with existing platforms like customer relationship management (CRM) software, enterprise resource planning (ERP) systems, and data analytics tools.
- Hardware Compatibility: AI tools, especially those relying on machine learning and deep learning, require significant computational power. It’s crucial to ensure that the hardware infrastructure supports these tools without overloading the system.
- Data Compatibility: AI tools should be able to process and analyze data from existing data sources, such as databases, data lakes, and real-time data streams.
- Scalability and Flexibility: AI solutions must scale with the organization’s growing demands and support flexible integrations with new technologies that may be introduced in the future.
1.2 The Risks of Choosing Incompatible AI Tools
Selecting AI tools without considering compatibility can lead to:
- Increased Costs: Additional resources and time may be required to make the AI tools work with the existing stack, resulting in extra costs.
- Integration Challenges: Incompatible tools can lead to integration failures, impacting business continuity and efficiency.
- Data Fragmentation: Without compatibility, businesses risk creating data silos that prevent seamless data flow across systems, ultimately impairing decision-making.
- Operational Disruptions: Introducing incompatible AI solutions could disrupt current workflows and require employees to adjust to new, inefficient processes.
Section 2: Key Factors to Consider When Evaluating Compatibility
When selecting AI tools, companies must assess various factors that determine how well the tools will integrate with their existing technology stack. These factors include:
2.1 Infrastructure and Hardware Compatibility
AI applications, particularly those involving machine learning (ML) and deep learning (DL), require substantial computational resources, including powerful CPUs, GPUs, and large memory capacity.
- Cloud-Based vs. On-Premise Infrastructure: Companies must decide whether to opt for cloud-based AI tools or on-premise installations. Cloud-based tools offer flexibility and scalability, but organizations with stringent data security policies may prefer on-premise solutions.
- Hardware Requirements: AI solutions, especially those used for deep learning, require specialized hardware like GPUs for efficient model training. It’s important to assess whether the existing infrastructure can support these needs.
- Compatibility with Existing Platforms: Ensure that the AI tool integrates with existing hardware resources, such as IoT devices or edge computing infrastructure, to avoid bottlenecks in performance.
2.2 Software Integration and APIs
Most businesses operate with a complex ecosystem of software applications. Whether using legacy systems or modern platforms, AI tools need to integrate seamlessly with these systems. Key considerations include:
- API Support: AI tools must provide well-documented and easy-to-use APIs that allow integration with CRM systems, ERP, analytics platforms, and more.
- Interoperability with Databases: AI solutions need to integrate with existing databases, whether SQL-based (e.g., MySQL, PostgreSQL) or NoSQL (e.g., MongoDB). It’s essential to ensure that data extraction, transformation, and loading (ETL) processes align with the AI tool’s requirements.
- Compatibility with Development Frameworks: Developers must check if the AI tool supports the programming languages and frameworks already in use within the organization, such as Python, Java, or R.
2.3 Data Compatibility and Management
Data is at the core of AI applications, and ensuring compatibility between AI tools and existing data management systems is critical. This includes:
- Data Storage: AI tools need to work seamlessly with existing data storage solutions, whether on-premise or in the cloud. Companies should assess whether the AI tool supports the formats, structures, and types of data that they already use.
- Data Formats: AI tools often require structured data (e.g., tabular formats) or unstructured data (e.g., images, text). Companies need to ensure that their data sources are compatible with these formats or are easily convertible.
- Data Security and Privacy Compliance: AI tools should comply with relevant data privacy regulations (e.g., GDPR, CCPA). This means ensuring that the tool can secure sensitive data and provide access control mechanisms.
2.4 Scalability and Future Growth
A company’s technology stack should evolve as the business grows, and so should the AI tools it integrates. When evaluating AI tools, it’s essential to consider:
- Scalability: Will the AI tool handle increasing data volumes, user traffic, or complexity as the company grows? Scalability ensures that the AI system can grow alongside the organization’s expanding needs without performance degradation.
- Cloud and Hybrid Integration: Many businesses operate in hybrid cloud environments, and it’s crucial to choose AI tools that offer compatibility with multi-cloud infrastructures. This flexibility ensures the company can scale without locking into a specific cloud provider.
2.5 Vendor Ecosystem and Support
Selecting AI tools from a vendor that aligns with existing technology providers is a strategic decision. Key factors to consider include:
- Vendor Support: Does the AI vendor provide adequate technical support, documentation, and resources to help with integration? A responsive support team can significantly ease the adoption process.
- Vendor Ecosystem: Does the AI vendor integrate with other tools and platforms in your existing stack? A vendor with a strong ecosystem and a range of complementary tools will facilitate a smoother integration process.
- Customization and Flexibility: Evaluate whether the AI tool can be customized to fit specific business needs, especially for businesses with niche requirements.
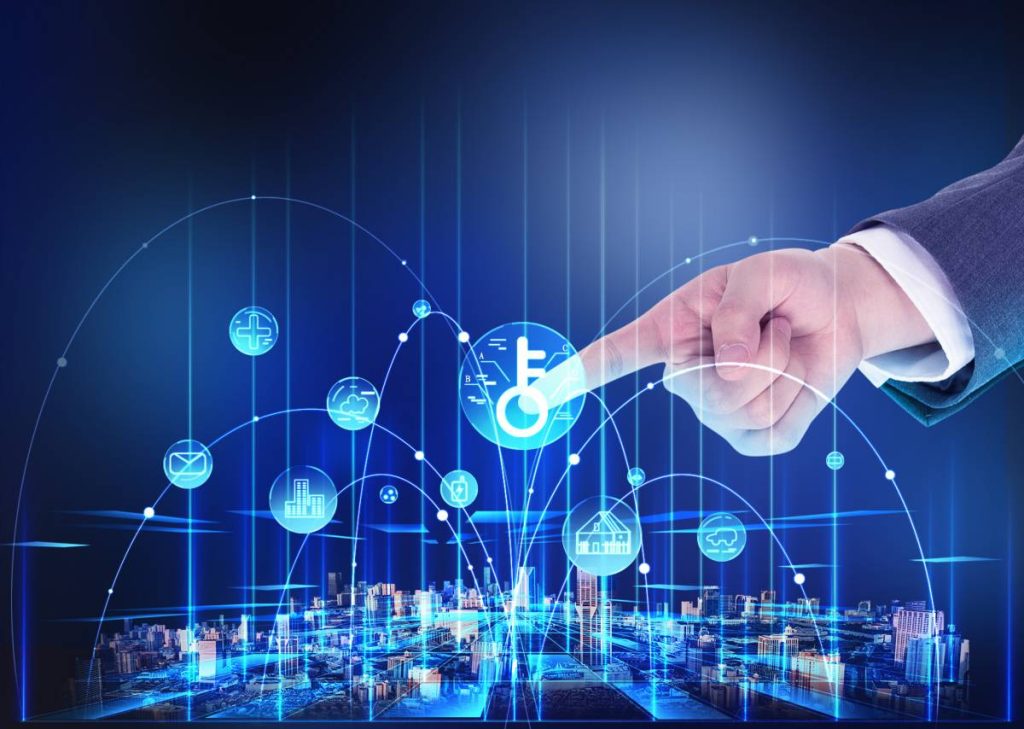
Section 3: Best Practices for Ensuring Compatibility with Existing Technology Stack
3.1 Conducting a Compatibility Assessment
Before selecting AI tools, businesses should conduct a thorough compatibility assessment that includes:
- A detailed review of the current technology stack, including software, hardware, databases, and infrastructure.
- Identifying specific business requirements and use cases that the AI tools need to address.
- Comparing the AI tool’s requirements (e.g., API support, hardware needs) against the existing infrastructure.
3.2 Collaborative Planning Between Teams
IT, data science, and business teams should collaborate when evaluating AI tools to ensure alignment between technical capabilities and business objectives. Cross-functional teams can provide valuable insights into integration challenges and potential roadblocks.
3.3 Proof of Concept (PoC) Testing
Before fully committing to a particular AI solution, organizations should run a Proof of Concept (PoC) with a small dataset to evaluate how well the AI tool integrates with existing systems. This allows businesses to assess its effectiveness, compatibility, and scalability.
3.4 Prioritize Open-Source and Flexible Tools
Choosing open-source AI tools or platforms that offer flexibility in terms of integration can help mitigate compatibility challenges. Open-source tools like TensorFlow, PyTorch, and Scikit-learn are highly customizable and can be adapted to a wide range of technology stacks.
Section 4: Real-World Case Studies
4.1 Case Study 1: Financial Services
A global financial institution was looking to implement AI-powered fraud detection tools. The company had a legacy CRM system and a database built on SQL. After assessing compatibility, they chose an AI tool that integrated seamlessly with SQL and provided robust API support for the CRM system. The successful integration reduced fraud detection time by 50% and improved accuracy.
4.2 Case Study 2: E-Commerce
An e-commerce business sought to use AI for personalized product recommendations. The company had a large database of customer interactions and a mix of cloud-based tools. After conducting a compatibility assessment, they chose an AI tool that integrated well with both cloud platforms and their existing recommendation engine, leading to a 20% increase in sales.
Conclusion
When selecting AI tools, businesses must prioritize compatibility with their existing technology stack to ensure smooth integration, scalability, and data security. By carefully evaluating factors such as infrastructure, data compatibility, scalability, and vendor ecosystem, companies can maximize the value of AI without disrupting existing systems. Following best practices like conducting compatibility assessments, involving cross-functional teams, and testing with PoCs can help businesses make well-informed decisions and achieve successful AI implementation.
By taking the time to align AI tools with their current tech stack, businesses can unlock the full potential of artificial intelligence while ensuring seamless, future-proof integrations.