Introduction:
The promise of autonomous driving—vehicles that can operate without human intervention—has captivated the imagination of consumers, automakers, and technologists for years. The idea of self-driving cars offers numerous benefits, including reduced traffic accidents, improved fuel efficiency, and a more convenient, efficient transportation system. Major companies such as Tesla, Google’s Waymo, Uber, and traditional automakers like Ford and General Motors have invested heavily in autonomous driving technology, and numerous advancements have been made in recent years.
However, despite the significant progress made, the question remains: How long until autonomous driving technology is fully commercialized and widely available to the public? While certain aspects of autonomous driving technology are already being tested on public roads, widespread adoption of self-driving cars will depend on a variety of factors, including technological advancements, regulatory approval, safety concerns, and public acceptance.
This article will explore the current state of autonomous driving technology, the obstacles that need to be overcome for full commercialization, and provide an estimate of when we might see fully autonomous vehicles on our roads.
1. Understanding Autonomous Driving: Levels of Automation
Before diving into the timeline for the full commercialization of autonomous driving technology, it’s important to understand the different levels of automation that exist, as defined by the Society of Automotive Engineers (SAE). These levels range from Level 0 (no automation) to Level 5 (full automation), each representing a different degree of vehicle autonomy.
- Level 0 (No Automation): The vehicle has no automated features. The driver is entirely responsible for all aspects of driving.
- Level 1 (Driver Assistance): Basic driver assistance systems, such as cruise control or lane-keeping assist, are available, but the driver is still responsible for most aspects of driving.
- Level 2 (Partial Automation): The vehicle can control both steering and acceleration/deceleration, but the driver must remain engaged and monitor the road. An example of this is Tesla’s Autopilot.
- Level 3 (Conditional Automation): The vehicle can perform most driving tasks in certain conditions, but the driver must be ready to intervene if necessary. Audi’s Traffic Jam Pilot is an example, though it’s only available in specific regions.
- Level 4 (High Automation): The vehicle can handle all driving tasks in certain conditions (such as within a specific geographic area or under specific circumstances) without human intervention. However, there may still be limitations, such as the vehicle not being able to operate in extreme weather.
- Level 5 (Full Automation): The vehicle is fully autonomous and can operate without any human intervention in all conditions and environments. No steering wheel, pedals, or human input is required.
Currently, most commercial vehicles on the road today are at Level 2 or Level 3 automation, with Level 4 and Level 5 still being developed and tested.
2. Technological Challenges to Full Commercialization
While there has been remarkable progress in the development of autonomous driving technology, achieving Level 5 autonomy—the point at which a vehicle can operate without any human intervention in all conditions—presents a range of technological challenges that need to be addressed.
2.1. Sensor and Perception Technologies
One of the critical technologies for autonomous vehicles is sensing and perception, which involves equipping the vehicle with a combination of cameras, radar, LiDAR (Light Detection and Ranging), and ultrasonic sensors to detect and interpret the surrounding environment. The car must be able to detect everything from pedestrians to traffic signs, other vehicles, animals, and even debris on the road.
Despite advancements in these sensors, challenges remain in ensuring that they can provide reliable and accurate data in all weather conditions and environments. For instance, LiDAR is often sensitive to rain, fog, or snow, which can cause it to malfunction. Similarly, cameras may struggle to identify objects in low-light conditions or glare from the sun.
To address these challenges, autonomous vehicles must rely on sensor fusion, where data from multiple sensors are combined to create a clearer picture of the environment. This fusion must be accurate and fast to make real-time driving decisions, which is particularly challenging in highly dynamic environments like city streets.
2.2. Artificial Intelligence and Machine Learning
At the heart of autonomous driving systems is artificial intelligence (AI), which is responsible for processing the vast amounts of data generated by sensors and making driving decisions. Machine learning (ML) models are trained on massive datasets of driving behavior, road conditions, and traffic scenarios to help the vehicle learn how to respond to various situations.
However, AI still has limitations when it comes to complex decision-making. For instance, autonomous vehicles must be able to handle edge cases—rare or unexpected situations that were not previously encountered during training. While AI can become very good at responding to routine scenarios, it can still struggle to make split-second decisions in more ambiguous or unusual situations.
Additionally, the need for real-time processing is critical. The vehicle’s AI must make decisions in a fraction of a second, ensuring that it can respond quickly to potential hazards. While modern processors are fast, the computing power required for this type of real-time decision-making can be immense and presents both hardware and software challenges.
2.3. Mapping and Navigation
Autonomous vehicles rely heavily on high-definition maps to navigate and understand their environment. These maps include detailed information about roadways, intersections, lane markings, and other critical infrastructure elements that the car needs to follow.
However, these maps must be continuously updated to account for changes in the environment, such as new construction, road closures, or altered traffic patterns. In addition, the vehicle’s sensors need to be able to interpret the environment in real-time, making the reliance on pre-loaded maps a complementary technology rather than the sole method of navigation.
Creating and maintaining high-definition maps for autonomous vehicles presents significant challenges, particularly when it comes to scaling them for use on a global level. The cost and logistics of mapping entire regions, especially in rural or remote areas, is a considerable barrier to widespread adoption.
2.4. Safety and Testing
Ensuring the safety of autonomous vehicles is paramount. The technology must be able to react to a wide range of driving scenarios and handle all potential risks, including unpredictable human drivers, pedestrians, cyclists, and various road hazards. Testing the technology in all potential real-world conditions—such as extreme weather, poor road conditions, or heavy traffic—presents a significant challenge.
Currently, autonomous vehicles undergo extensive testing, including simulation environments, controlled test tracks, and real-world road testing. However, there is still much to learn about how self-driving cars will perform in unpredictable, real-world environments.
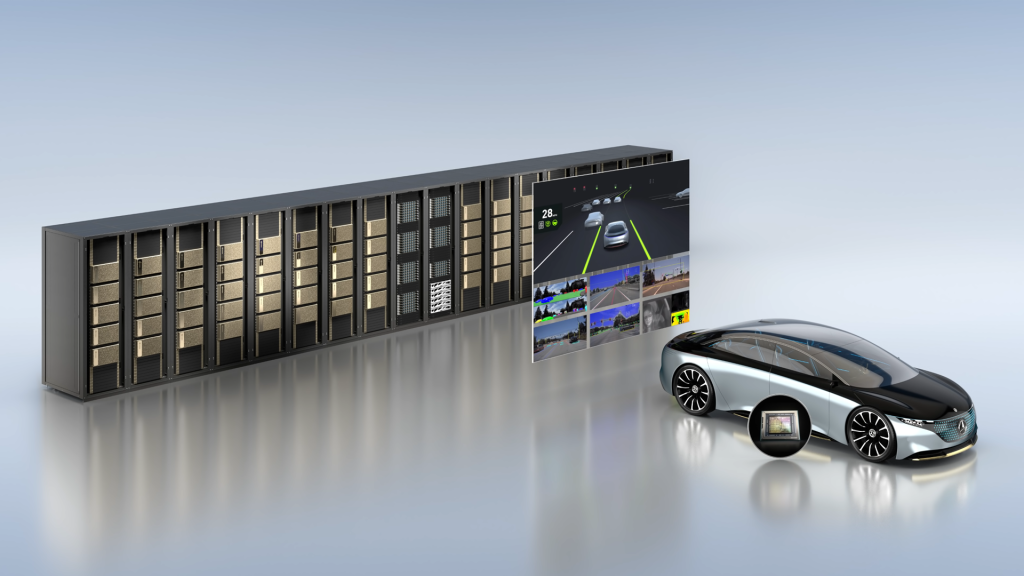
3. Regulatory and Legal Challenges
In addition to technological hurdles, regulatory and legal challenges will play a critical role in the timeline for the commercialization of autonomous vehicles. These challenges include:
3.1. Regulatory Approval
The deployment of autonomous vehicles will require new regulations and safety standards to be developed. Different countries have varying laws governing driverless cars, and international consistency is needed for a seamless rollout of autonomous vehicles. Regulators must ensure that autonomous cars meet safety standards, including how they interact with human drivers, pedestrians, and cyclists.
In the United States, for example, the National Highway Traffic Safety Administration (NHTSA) has made strides in issuing guidelines for autonomous vehicles, but there is no universal framework for how they will be regulated across all states. In Europe, the European Commission is also working to establish regulations for the safe deployment of autonomous vehicles. Harmonizing regulations across regions will be essential for companies to scale their operations globally.
3.2. Liability and Insurance
One of the most complex legal challenges surrounding autonomous vehicles is determining liability in the event of an accident. If a fully autonomous vehicle is involved in a crash, it may not be immediately clear whether the fault lies with the vehicle’s AI, the human driver (if any), or other external factors. Establishing clear liability frameworks for autonomous vehicles will be critical for both legal clarity and the insurance industry.
Insurance companies will need to adapt to this new landscape, creating policies that cover both human and machine liability. Furthermore, vehicle manufacturers and technology providers may need to take on more responsibility for ensuring their systems are fail-safe, especially when it comes to accidents caused by AI errors.
3.3. Public Trust and Acceptance
For autonomous driving technology to be successfully commercialized, public trust and acceptance are essential. Consumers will need to feel confident that self-driving cars are safe and reliable, and there may be resistance to fully autonomous vehicles if people are hesitant to relinquish control. Additionally, the public needs to be educated on the benefits of autonomous driving, such as reduced accidents, less congestion, and greater mobility for disabled individuals.
As autonomous vehicles become more common, manufacturers will need to address concerns about cybersecurity, data privacy, and transparency to ensure that users feel secure in using the technology. Gradually, as people become more familiar with autonomous driving systems and witness their effectiveness in controlled environments, the level of trust in the technology will increase.
4. Timeline for Full Commercialization
While it is difficult to pinpoint an exact date for when autonomous driving technology will be fully commercialized, most industry experts agree that Level 5 autonomous vehicles are still a few years—or even decades—away. Here’s a rough timeline of what we can expect:
- 2025-2030: Expect to see widespread Level 3 and Level 4 autonomous vehicles on the road. These vehicles will operate in specific areas or under specific conditions, such as in geo-fenced zones or during low-traffic conditions. These cars will still require human intervention in certain scenarios but will be capable of handling many routine driving tasks autonomously.
- 2030-2040: Full Level 5 autonomous vehicles could start to enter commercial markets, especially in controlled environments like urban centers or designated highways. However, issues such as regulatory approval, infrastructure changes, and public trust may continue to slow widespread adoption.
- 2040 and Beyond: Full commercialization and widespread adoption of Level 5 autonomous vehicles could become a reality, transforming the global transportation system. By this time, most vehicles may be fully autonomous, with human-driven cars becoming increasingly rare.
Conclusion:
Autonomous driving technology is advancing rapidly, and while fully self-driving cars are not yet widely available, significant progress is being made toward their commercialization. Current technology, such as Level 3 and Level 4 autonomous systems, will likely become more commonplace in the next few years. However, fully autonomous vehicles that require no human intervention—Level 5—will take longer to perfect and deploy.
The widespread adoption of autonomous driving will depend on overcoming a variety of challenges, including technological improvements, regulatory frameworks, safety concerns, and public acceptance. While we may not see fully autonomous vehicles on all roads in the near future, the ongoing advancements and investment in the field suggest that self-driving cars could become a mainstream reality by the 2030s or 2040s.